Reformation’s Vertical AI Partner, XGEN AI, Comes Out of Stealth
Another artificial intelligence-based startup has emerged.
XGEN AI, a vertical AI integrator that offers a slew of options for composable commerce, announced its launch Tuesday. Though its business has only just started to take root officially, the New York-based startup already has signed a number of partners.
More from Sourcing Journal
The company has joined forces with Reformation, Valentino, MSGM and other fashion and luxury apparel clients to create customizable AI systems that take a few hours—rather than weeks or months—to train.
When Frank Faricy, the founder and CEO of the company, began building XGEN AI, he said he wanted to address the gap he saw between technology teams and end users inside organizations. Because AI models—and other technology tools—can be difficult to build and implement, he said, sometimes internal teams lack communication until it’s time for adoption of a new technology to begin.
“AI teams don’t get e-commerce teams and vice versa,” he said. “The only way we’re going to see actual AI adoption in [the retail] space—or in any space—is horizontal AI applied in a vertical way.”
Verticalized AI refers to systems designed for highly specific functionalities. They require less time spent training, because the models have to ingest less data than a generalized AI system—particularly a generalized large language model—would.
Each of XGEN AI’s products, Faricy said, can be both configured and used by employees who don’t primarily focus on technology in their day-to-day work.
“For e-commerce teams and digital teams, AI is coming…and I think people need to recognize that and become the local experts,” Faricy explained. “If you are [on] an e-comm team, but you’re also the AI expert—you’re literally telling your managers, ‘Yeah, I’m deploying different AI models on a daily basis.’ They’re going to be like, ‘This person is gold.’”
The startup has already begun offering three core products, which it calls XSearch, XRecommend and XGenerate. Each of the products consists of several AI “plugins,” so clients can determine which pieces of the product they want to use, based on their business goals.
“A product to us is a group of models, data integrations and microservices,” Faricy said.
Companies can tweak the functions to their direct needs with the help of the XGEN AI team, which has been backed by AWS and exhibited with the cloud giant at the National Retail Federation’s conference in January.
Faricy noted that XGEN AI has plans to release six more products this year. One will be called XVision; it will use computer vision for dozens of different tasks. Another will be a chatbot composer, which Faricy said some clients have already begun testing.
“One of our customers testing it right now said, ‘Hey, half of our traffic is support pages—can the chatbot answer this?’” Faricy told Sourcing Journal. “They uploaded the support articles, put that into that plugin, and then all of a sudden the chatbot is answering all sorts of product-related questions, support questions.”
Though many companies have started using chatbots for FAQs, Faricy said the new product will have a broad array of applications that can be customized to each client’s direct needs.
Faricy did not disclose the functionalities of the other four products coming in 2024.
The startup has already raised more than $16 million in pre-seed and seed funding. Faricy said the company has lofty goals for the remainder of 2024. He noted the team will double in size this year, and that the company will look to scale revenue to eight figures in 2024.
As conversations about AI continue to swirl, one main focus among potential practitioners has been data. The quality of the data used to train a model has a significant impact on the caliber of the output. But for many companies, cleaning their data and identifying which data points they should be training models on has proven a gargantuan task.
Faricy said that while better data equals better results, XGEN AI’s products can meet a company where they are.
“I recognized early on that the data problem is massive in this vertical. So it’s the non-variable in the equation,” Faricy said. “It doesn’t matter how bad your data is. Every model in the ecosystem will work with what you’ve got.”
Solve the daily Crossword

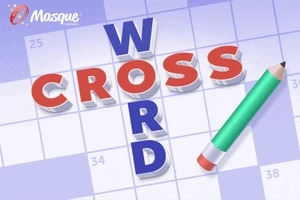